The term “data science” seems to be on everyone’s lips these days, but have you ever wondered who actually invented this discipline? Is it a modern invention, or does its origin trace back further than we think?
Data science, as a formal field, doesn’t have a single inventor. It evolved over time, drawing from statistics, computer science, information theory, and other domains. However, the term “Data Science” was coined by William S. Cleveland in 2001 to describe an expansion of statistics into broader areas of expertise.
Stay tuned as we dive deeper into the history, evolution, and key figures that have shaped what we know today as data science.
The Evolutionary Path: From Statistics to Data Science
Data science is an amalgamation of various disciplines. It’s not a field that sprang up overnight but evolved over decades, pulling in techniques from statistics, machine learning, and computer science.
William S. Cleveland: Coining the Term
In 2001, statistician William S. Cleveland introduced the term “Data Science” in his paper to advocate for an expansion of statistics beyond theory into technical areas. This was a pivotal moment in defining the field.
The Big Data Era: A Paradigm Shift
The advent of Big Data has been another significant milestone, bringing about new challenges and opportunities. This ushered in the need for more advanced tools and methodologies, further evolving the field.
Key Figures in Data Science History
Discuss other influential people who have contributed to the development and popularization of data science.
Data Science vs. Statistics: A Detailed Comparison
Examine how data science goes beyond traditional statistics and incorporates other disciplines like machine learning and business analytics.
The Modern Landscape: Data Science Today
Provide an overview of what data science looks like today, including its applications, tools, and significance.
The question of who invented data science doesn’t have a straightforward answer. It’s a discipline that has grown organically over time, incorporating various other fields like statistics, machine learning, and computer science. While William S. Cleveland can be credited with coining the term “Data Science,” the field itself is a collective effort of many brilliant minds and evolving technologies. Today, data science stands as an interdisciplinary field with immense potential, offering insights that range from business decision-making to groundbreaking scientific research. As we continue to generate more data, the importance and scope of data science are only likely to grow.
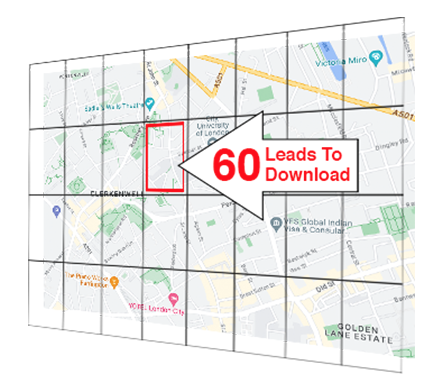
90 Free Leads When You Register!
Find accurate B2B contact information for targeted marketing. Close more deals and start more conversations.
The Role Of Data Journalism In Popularizing Data Science
As data science has evolved, so has its visibility in the public sphere. One conduit for this visibility is data journalism. But what role has data journalism played in making data science a household term?
Data journalism has been pivotal in bringing the concepts and techniques of data science to the mainstream. By using data science methods to analyze and interpret data for storytelling, data journalism has demystified complex topics and made them accessible to the general public.
Want to understand the symbiotic relationship between data journalism and data science? Keep reading as we explore how each enriches the other and collectively contributes to the broader understanding of data.
Spreading Awareness: Data Journalism as a Catalyst
Data journalism employs data science methods to investigate issues, create compelling narratives, and offer insights that wouldn’t be apparent through conventional reporting. This practice has served to introduce a broad audience to the utility and significance of data science.
Mutually Beneficial: A Two-Way Street
Not only does data journalism utilize data science techniques, but the relationship is also reciprocal. As data journalism has grown in prominence, it has inspired data scientists to develop new methods and tools that aid journalistic investigations.
Case Studies: Data Journalism in Action
Data journalism has been at the forefront of uncovering crucial societal issues, from political scandals to public health crises, further demonstrating the importance and versatility of data science.
Tools and Techniques: Shared Arsenal
Discuss the common tools and techniques used in both data journalism and data science, emphasizing the overlap.
Impactful Stories: Milestones in Data Journalism
Highlight significant stories where data journalism made a meaningful impact, thereby showcasing the power of data science.
Challenges and Ethical Considerations
Discuss the ethical implications and challenges that arise when data journalism employs data science methodologies.
Data journalism has been instrumental in elevating the public’s understanding of data science. This symbiotic relationship has not only made data science more accessible but also spurred advancements in both fields. As we navigate an increasingly data-driven world, the marriage between data journalism and data science will likely become even more integral, shaping how we consume information and make sense of the world around us.
Academic Contributions: How Universities and Research Institutions Shaped Data Science
While the corporate world and journalism have had their impact, academia deserves a special mention in the evolution of data science. What role have universities and research institutions played in the development and popularization of this field?
Universities and research institutions have been central to the development of data science, providing rigorous academic frameworks and research opportunities. Through curricula, publications, and high-impact research, academia has legitimized data science as a distinct field of study and contributed to its advancement.
Intrigued by how the ivory towers have influenced this multidisciplinary field? Continue reading to discover specific contributions, key academic figures, and how academia continues to shape the future of data science.
Curricular Developments: The Birth of Data Science Programs
Universities have responded to the growing demand for data science expertise by launching specialized courses and degree programs. These programs offer comprehensive training, covering everything from statistics and machine learning to ethics and business applications.
Research Contributions: Pioneering Work
Academic research has often been at the forefront of data science innovations. Whether it’s developing new algorithms, exploring novel applications, or studying ethical implications, academia serves as a hub for cutting-edge research.
Key Academic Figures: The Thought Leaders
From statisticians to computer scientists, several academics have made significant contributions to data science. Their work not only shapes academic discourse but also has practical applications that benefit society at large.
Evolution of Data Science Curriculum
Discuss how data science programs at universities have evolved over the years, adapting to new technologies and methodologies.
Landmark Research Papers in Data Science
Highlight key academic papers that have been seminal in shaping the field of data science.
Collaborations Between Academia and Industry
Examine how academic institutions are partnering with businesses to drive data science innovations.
Universities and research institutions have played an indelible role in shaping data science into what it is today. Through education and research, they’ve not only developed the intellectual foundation for the field but have also provided a launchpad for practical innovations and applications. As the field of data science continues to grow and evolve, academia will undoubtedly remain a key player in its ongoing maturation and refinement.
Government Initiatives: The Public Sector’s Role in Advancing Data Science
Beyond academia and journalism, government initiatives have had a significant impact on the data science landscape. But how exactly has the public sector contributed to the advancement of this discipline?
Government agencies have been instrumental in advancing data science by funding research, implementing data-driven decision-making, and even creating specialized units for data analysis. These initiatives not only bolster national capabilities but also set standards that can influence data science practices globally.
Interested in learning how governments are not just consumers but also drivers of data science innovation? Let’s delve into the specific initiatives, funding avenues, and collaborations that spotlight the government’s role in this field.
Funding and Grants: The Financial Backbone
Governments often allocate significant budgets for research and development in data science. These funds enable both academic and independent researchers to pursue groundbreaking work, leading to new technologies and methodologies.
Policy Frameworks: Setting the Stage
Public sector involvement also extends to policy-making, where governments can set ethical and quality standards for data science projects. Regulatory frameworks can thus serve to shape the field responsibly.
Collaborations and Partnerships: United We Stand
Many governments engage in collaborations with the private sector and academia, creating a synergistic environment where data science can thrive. These partnerships often result in public data sets, shared resources, and joint research projects.
Case Studies: Government-led Data Science Projects
Highlight successful data science projects initiated by governments, showing their impact on society.
Regulatory Challenges and Solutions
Discuss the difficulties governments face when regulating this fast-evolving field, and how they are addressing these challenges.
Future Prospects: Government’s Role in Data Science
Explore what the future holds in terms of government involvement in data science, including emerging trends and potential new initiatives.
The role of government in advancing data science is multi-faceted and incredibly significant. From funding and policy-making to partnerships, public sector involvement serves as a cornerstone for the field’s growth and ethical considerations. As data science continues to impact various aspects of society, the synergies between government and this discipline are set to become increasingly crucial.
The Business Boom: Corporate Influence in the Progress of Data Science
We’ve examined the roles of academia and government, but what about the corporate world? How have businesses influenced the development and proliferation of data science?
Businesses have been pivotal in applying data science to solve real-world problems, driving its commercialization and making it indispensable in various industries. From healthcare and finance to marketing and logistics, corporate involvement has made data science not just an academic concept but a practical tool for innovation.
Curious about how Fortune 500 companies or even startups have contributed to data science? Stick around as we discuss groundbreaking corporate projects, key industry players, and the future of data science in the business realm.
Commercial Applications: Practical Solutions
Companies have led the way in showing how data science can address specific challenges. These commercial applications serve as compelling case studies and also inspire academic research.
Talent and Skill Development: Nurturing the Next Generation
Businesses often collaborate with educational institutions for training programs, further bridging the gap between academia and practical application. Corporate demand for data science talent also shapes the curricula and focus of academic courses.
Innovation and Entrepreneurship: Fostering Growth
Startups and established firms alike have been incubators for data science innovation. Their risk-taking and agility often lead to the development of new techniques and technologies that push the field forward.
Sector-Specific Impacts of Data Science
Discuss how data science has affected different sectors such as healthcare, finance, and manufacturing.
Corporate Data Science Ethics
Explore the ethical considerations that companies face when implementing data science projects.
Key Business Leaders in Data Science
Profile industry leaders who have been instrumental in making significant contributions to the field.
The business sector has been a driving force in bringing data science from the theoretical to the practical, shaping its applications and contributing to its rapid advancement. Corporate involvement complements the roles played by academia and government, filling gaps and creating a more rounded and robust data science ecosystem. As industries continue to adapt and innovate, their influence on the trajectory of data science is certain to remain significant.
The Societal Impact: How Data Science Affects Everyday Lives
We’ve talked about academia, government, and businesses, but what about the average person? How has the emergence and growth of data science impacted society at large?
Data science has had a profound societal impact by revolutionizing how we live, work, and even think. From personalized healthcare and optimized public transportation to recommendation engines in shopping and entertainment, data science touches almost every facet of our daily lives.
Want to know how data science is shaping your daily experiences and the world around you? Let’s explore the transformative societal impacts that make data science more than just a buzzword.
Personalized Experiences: Catering to Individual Needs
Data science algorithms drive the personalized experiences we’ve come to expect in everything from online shopping to content streaming services. This has shifted consumer expectations and created new business models centered around individual preferences.
Public Services: Elevating Quality of Life
Data science has also found its way into public services like healthcare, transportation, and urban planning. The analytics and predictive models improve efficiency, resource allocation, and overall service quality.
Social Change: Data-Driven Advocacy
Nonprofits and activists are employing data science to highlight social issues and advocate for change. The data-driven approach brings a new level of objectivity and effectiveness to their campaigns.
Data Privacy Concerns
Delve into the challenges and concerns surrounding data privacy and what is being done to address them.
The Digital Divide: Access to Data Science
Examine the inequality in access to data science technology and education, and its societal implications.
The Future of Data Science in Society
Discuss what the future holds for the intersection of data science and society, including emerging trends and potential risks.
Data science has indeed become a cornerstone of modern society, influencing various aspects of our lives, from personal experiences to social change. Its omnipresence signifies its importance, but also calls for responsible usage and ethical considerations. As data science technologies continue to evolve, their role in shaping societal norms and experiences will only grow, making this an area worth watching and understanding.
The Ethical Frontier: Navigating Moral Dilemmas in Data Science
We’ve covered multiple facets of data science, but there’s one crucial aspect that cannot be ignored: ethics. How are ethical concerns being addressed in this rapidly evolving field?
Ethics in data science is not merely an afterthought; it’s an integral part of responsible research and application. Issues like data privacy, consent, and algorithmic fairness are central to the discourse, aimed at ensuring that data science serves the broader good without compromising individual rights or perpetuating biases.
If you’re interested in the intersection of technology and morality, don’t go away. We’re about to dive deep into the ethical questions that data science raises and the guidelines that are emerging to navigate them.
Data Privacy: A Fundamental Right
Data privacy is at the forefront of ethical considerations. The massive collection and analysis of data need to be counterbalanced by strong privacy measures to protect individuals’ information.
Algorithmic Fairness: Equal Representation
Bias in data or algorithms can have harmful consequences, such as perpetuating societal inequalities. Therefore, developing equitable algorithms is a moral imperative in data science.
Informed Consent: Power to the People
Acquiring explicit consent from data subjects, especially in sensitive fields like healthcare, is vital. This ensures that individuals are aware of how their data is being used and are in control of their personal information.
Emerging Ethical Guidelines
Discuss the development of ethical guidelines and standards for data science, both within organizations and at the broader societal level.
Case Studies: Ethical Dilemmas and Resolutions
Present real-life examples that showcase how ethical issues have been handled in various data science projects.
Regulatory Landscape: Legal Frameworks for Data Science Ethics
Explore the existing and upcoming regulations aimed at governing the ethical use of data science.
Ethics in data science is a complex but essential area that complements its technical advancements. As we navigate the future of this burgeoning field, understanding and integrating ethical considerations become not just advisable but imperative. By fostering an ethical approach, data science can better serve society, minimize harm, and pave the way for innovations that benefit all.
The Global Landscape: How Different Countries Approach Data Science
So far, we’ve explored the academic, commercial, and ethical dimensions of data science. But what does the global landscape look like? How are different countries adopting and adapting to this transformative field?
The global landscape for data science is diverse and dynamic, with countries taking various approaches based on their economic needs, technological capabilities, and societal values. While nations like the United States and China are leading in terms of innovation and scale, smaller countries are also making significant contributions, especially in specialized areas like healthcare analytics and environmental monitoring.
Curious to learn about how data science manifests around the world? We’ll take a whirlwind tour of different countries’ approaches, highlighting key players, emerging trends, and unique contributions.
The United States: A Hub of Innovation
Home to Silicon Valley, the United States remains at the forefront of data science innovation, driving both technological breakthroughs and academic research.
China: Scale and Scope
With its robust tech industry and vast datasets, China is a key player in data science, especially in areas like AI, machine learning, and big data analytics.
European Union: Pioneers in Data Ethics
The EU, with its General Data Protection Regulation (GDPR), has taken the lead in setting global standards for data privacy and ethics.
Emerging Markets: Untapped Potential
Countries like India and Brazil are rapidly evolving in the field of data science, with an increasing focus on education, start-ups, and public-private partnerships.
Country-Specific Challenges
Discuss the unique challenges that different countries face in adopting data science, including regulatory hurdles and skills gaps.
Cross-Border Collaborations
Explore how international partnerships and collaborations are enriching the field of data science.
Global Data Science Education
Look at how different educational systems across the world are incorporating data science into their curricula and the impact it’s having.
Data science is indeed a global phenomenon, shaping and being shaped by various cultures, economies, and regulatory landscapes. As countries learn from each other’s successes and failures, the field becomes more enriched and capable of addressing a broader array of challenges. Understanding these global dynamics is key for anyone interested in the future of data science, as it transcends borders and promises to be a major force in shaping our collective future.
Conclusion
The realm of data science is as expansive as it is impactful, touching multiple facets of society, technology, and even ethics. As we’ve navigated through its origins, ethical implications, and the diverse approaches adopted globally, it’s evident that data science is more than a mere buzzword—it’s a multidisciplinary field that requires deep understanding and responsible application. Whether you’re a seasoned professional, an academic, or a curious observer, comprehending these intricate layers will equip you to engage meaningfully with data science.
As countries and communities collaborate and innovate, the field promises to evolve, raising new questions and offering unprecedented solutions. In this ever-changing landscape, staying informed and ethically grounded is not just beneficial—it’s imperative for the greater good.
References
https://en.wikipedia.org/wiki/Data_science
https://www.prospects.ac.uk/job-profiles/data-scientist
https://www.oracle.com/uk/what-is-data-science/