Data analysis is at the heart of many scientific, financial, and social decisions. But where did this indispensable tool originate, and who were the pioneers behind its development?
The invention of data analysis can’t be attributed to a single individual or moment. It evolved over time, drawing from statistics, mathematics, and computer science. Early contributors include Ronald A. Fisher in statistics, John Tukey in exploratory data analysis, and more recently, Hadley Wickham in the realm of data science with tools like ggplot2 for R.
Eager to understand how these pioneers contributed to the field of data analysis? Let’s delve into their groundbreaking work and how it laid the foundation for modern data analysis.
Ronald A. Fisher and Statistics
Fisher was an early pioneer in the field of statistics, and his work laid the foundation for data analysis. His techniques, like variance analysis and linear regression, are still widely used today.
John Tukey and Exploratory Data Analysis
John Tukey coined the term “exploratory data analysis” and emphasized the importance of understanding data before making hypotheses. He developed techniques like box plots that are still in use today.
Hadley Wickham and Modern Data Science
Wickham is known for his contributions to data visualization through tools like ggplot2 in R. His work has modernized data analysis and made it more accessible.
Transition from Paper to Computers
Early data analysis was carried out on paper or simple computing machines, but the advent of modern computers has greatly expanded its scope and depth.
Interdisciplinary Influence
Data analysis has been influenced by multiple disciplines, including economics, sociology, and even psychology. This has enriched the field and widened its applications.
Future Trends in Data Analysis
With the growth of big data and machine learning, data analysis is continually evolving, offering exciting opportunities and challenges.
The invention of data analysis is a collective achievement, contributed to by pioneers from diverse fields like statistics, computer science, and even social sciences. From Ronald A. Fisher’s foundational work in statistics to John Tukey’s exploratory techniques and Hadley Wickham’s contributions to modern data science, the field has continually evolved to become an indispensable tool in our data-driven world. As technologies and methodologies advance, data analysis promises to become even more vital, serving as a crucial lens through which we understand and engage with the world around us.
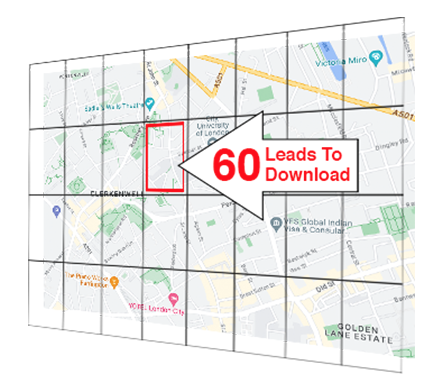
90 Free Leads When You Register!
Find accurate B2B contact information for targeted marketing. Close more deals and start more conversations.
The Impact of Data Analysis on Various Industries
While we’ve delved into the historical origins of data analysis, its modern implications are just as compelling. Data analysis is a versatile tool that has made significant inroads into various industries. But how exactly has it shaped these fields?
Data analysis has profoundly impacted a range of industries, including healthcare, finance, marketing, and more. It has enabled data-driven decision-making, predictive modeling, and real-time analytics, transforming the way these sectors operate.
Curious to know the specific ways data analysis has revolutionized different industries? Let’s dive deeper into the nuances of its influence on healthcare, finance, and other sectors.
Healthcare
Data analysis in healthcare has revolutionized patient care and treatment plans. It is used to analyze medical records, improve diagnostics, and even predict disease outbreaks.
Finance
In finance, data analysis is used for risk assessment, portfolio management, and fraud detection. It has significantly reduced the margin of error and increased efficiency.
Marketing
In the realm of marketing, data analysis helps in customer segmentation, targeted advertising, and measuring the ROI of various marketing campaigns.
Transportation and Logistics
Data analysis is helping optimize routes, reduce fuel consumption, and enhance supply chain management in the transportation sector.
Environmental Studies
By analyzing climate data, scientists are making more accurate predictions about weather patterns and global warming, thereby informing policy decisions.
Real Estate
In real estate, data analysis assists in price estimation, market trend prediction, and even identifying potential areas for development.
The influence of data analysis extends far beyond its academic or tech-oriented beginnings. Today, it serves as a cornerstone in a myriad of industries, each leveraging its capabilities to optimize performance, streamline processes, and make more informed decisions. Whether it’s predicting stock market trends, diagnosing medical conditions, or optimizing marketing strategies, data analysis has established itself as an invaluable asset across the board. As technology continues to advance, the applications and significance of data analysis are only expected to grow, further solidifying its role as a transformative force in the modern world.
Ethical Considerations in the Application of Data Analysis
As data analysis becomes more integrated into various sectors, it raises critical ethical questions. What are the ethical implications of data collection and analysis, and how can they be responsibly addressed?
Ethical considerations in data analysis include issues like data privacy, informed consent, and algorithmic bias. Addressing these responsibly involves transparent data collection methods, ensuring user consent, and periodic reviews to identify and mitigate biases in data-driven systems.
Intrigued by the ethical dilemmas that come with the power of data analysis? Continue reading to explore the complexities of responsibly managing data in an increasingly digital world.
Data Privacy
One of the major ethical concerns is data privacy. Users need assurance that their data won’t be misused or exposed. Implementing strong encryption methods and having a transparent data usage policy are vital steps.
Informed Consent
Another ethical issue is obtaining informed consent from individuals whose data is being collected and analyzed. This is especially critical in fields like healthcare and social research.
Algorithmic Bias
Algorithms used in data analysis can inadvertently include biases, affecting the quality and fairness of insights. Periodic audits can help to identify and correct such biases.
Regulations and Compliance
Various jurisdictions have introduced regulations like GDPR in Europe and CCPA in California to safeguard users’ data.
Ethical Committees and Oversight
Some organizations and research institutions have ethical committees to review data collection and analysis methods, ensuring they meet ethical standards.
Long-term Ethical Implications
The ethical considerations are not static; they evolve with technology. Ongoing conversations and revisions to ethical guidelines are needed to stay up-to-date.
As we wield the power of data analysis to transform industries and improve lives, it’s imperative not to overlook the ethical dimensions that come with it. From safeguarding individual privacy to eliminating algorithmic biases, ethical considerations are integral to responsible data usage. Regulations and oversight committees offer some level of protection, but the long-term solution lies in the continual reevaluation and adaptation of ethical practices. It’s not just about what data analysis can do; it’s also about what it should do, ethically speaking, in an ever-evolving digital landscape.
The Future of Data Analysis: Emerging Trends and Technologies
The world of data analysis is not static; it is continuously evolving, thanks to rapid technological advancements. So what can we expect from the future of data analysis, and what emerging trends should we be looking out for?
The future of data analysis is geared towards automation, real-time analytics, and integration with artificial intelligence and machine learning. These advancements are set to revolutionize the speed, accuracy, and breadth of data interpretation, paving the way for more sophisticated decision-making processes.
Want to know more about how technologies like machine learning and AI will further shape the world of data analysis? Let’s explore these exciting future trends in greater detail.
Automation in Data Analysis
Automation tools are reducing the manual labor involved in data cleaning and preparation, making the analysis process more efficient and less prone to human error.
Real-Time Analytics
The ability to analyze data in real-time is becoming increasingly crucial, especially for industries like finance and healthcare where instant decision-making can be vital.
AI and Machine Learning Integration
Artificial Intelligence and Machine Learning are expected to play a significant role in automating complex data analysis tasks, including predictive analytics and natural language processing.
Quantum Computing and Data Analysis
Quantum computing promises to exponentially speed up data processing, which could bring about a new era in data analysis capabilities.
Ethics and Future Technologies
As technology evolves, ethical considerations will also need to adapt. New tech will likely introduce new ethical dilemmas that will need to be addressed.
User Experience and Data Visualization
Improvements in data visualization tools are making it easier for non-experts to understand complex data, which is a boon for democratizing data analysis.
The future of data analysis looks incredibly promising, with technological advancements expected to revolutionize how we collect, analyze, and interpret data. Automation, real-time analytics, and AI integration are just the tip of the iceberg. As we move into this future, it’s also essential to adapt our ethical frameworks and be prepared for the new challenges and opportunities that these advancements will undoubtedly bring. By keeping an eye on these emerging trends, we can better anticipate the needs of tomorrow and fully harness the power of data analysis for a more informed and efficient world.
Practical Applications: Case Studies of Data Analysis in Action
You may be wondering how the intricate techniques of data analysis actually manifest in real-world scenarios. Can you think of any groundbreaking case studies that effectively demonstrate the power of data analysis?
Indeed, data analysis has been at the forefront of several groundbreaking initiatives. For instance, it played a key role in optimizing Netflix’s content recommendation system, improving customer satisfaction and engagement. Additionally, it has been instrumental in public health initiatives, such as tracking and mitigating the spread of infectious diseases.
Ready for an eye-opening journey through some of the most compelling case studies where data analysis made a significant impact? Let’s take a closer look at how it’s shaping everything from entertainment to public health.
Netflix and Content Recommendation
Netflix uses intricate data analysis algorithms to understand viewer preferences. This has significantly improved its content recommendation system, resulting in increased viewer engagement and subscription retention.
Public Health and Disease Tracking
Data analysis has been invaluable in tracking the spread of diseases like COVID-19. Analyzing infection rates, mobility data, and public behavior has helped policymakers make informed decisions.
Retail and Customer Experience
Companies like Amazon use data analysis to understand customer buying patterns, optimize inventory, and provide personalized shopping experiences.
Sports Analytics
Data analysis is revolutionizing how athletes train and how games are strategized, offering insights into player performance, injury risks, and more.
Energy Sector
In the energy sector, data analysis helps in optimizing grid distribution, predicting equipment failure, and implementing sustainable energy solutions.
Smart Cities
Cities are becoming smarter with the help of data analysis, from optimizing traffic flow to reducing energy consumption in public spaces.
Real-world case studies provide undeniable evidence of data analysis’s transformative power. From enhancing viewer experiences on Netflix to tracking global pandemics and improving customer experiences in retail, data analysis is more than just a collection of techniques; it’s a catalyst for meaningful change across various sectors. These case studies not only illustrate its practical utility but also underscore its ever-increasing importance in our data-driven world. Whether we’re aware of it or not, data analysis impacts our lives daily and promises to continue shaping our future in ways we can only begin to imagine.
Navigating Challenges: The Roadblocks in Data Analysis
While data analysis holds enormous potential, it’s essential to acknowledge the challenges that professionals and organizations face in implementing it effectively. What are the typical roadblocks, and how can they be overcome?
Some common challenges in data analysis include data quality issues, lack of skilled personnel, and data security concerns. Addressing these roadblocks usually involves investing in proper data cleaning protocols, training or hiring skilled analysts, and implementing robust security measures.
Eager to delve deeper into these challenges and explore strategies for overcoming them? Let’s unpack the intricacies involved in navigating the roadblocks in data analysis.
Data Quality
Poor data quality, such as missing or inconsistent data, can significantly impact the analysis. Cleaning the data and ensuring its integrity are critical initial steps to obtain reliable results.
Lack of Skilled Personnel
The dearth of adequately trained analysts can hinder data analysis projects. Investing in training programs or hiring skilled analysts can address this issue.
Data Security Concerns
Data breaches and unauthorized access can compromise sensitive information. Implementing robust security protocols, like encryption and two-factor authentication, can mitigate these risks.
Scalability Issues
As data sets grow, maintaining the same level of analysis quality becomes challenging. Advanced tools and cloud-based solutions can help in scaling the data analysis operations.
Interoperability
Using multiple data sources can lead to compatibility issues. Adopting standardized formats and APIs can solve these problems.
Regulatory Challenges
Compliance with regional and industry-specific data regulations, like GDPR, can be a complex task. Regular audits and legal consultations are advised to ensure compliance.
While the challenges in data analysis are significant, they are not insurmountable. From maintaining data quality to ensuring its security and compliance, each roadblock has viable solutions that organizations can implement. Recognizing these challenges is the first step towards developing effective strategies to overcome them. As data analysis continues to evolve, staying ahead of these challenges becomes not just an option but a necessity for organizations aiming to leverage the full potential of their data.
Educational Pathways: How to Become Proficient in Data Analysis
Given the immense importance and scope of data analysis, you might be wondering what educational pathways can lead to proficiency in this field. Are there specific courses, degrees, or certifications that one should aim for?
To become proficient in data analysis, one typically needs a strong foundation in mathematics and statistics, followed by specialized training in data analysis techniques. This can be achieved through a bachelor’s degree in a related field, and often, a master’s degree for advanced roles. Certifications in specific software or methodologies are also beneficial.
Interested in diving deeper into the educational routes that can make you a data analysis expert? Let’s explore the available options in more detail, from traditional degree programs to modern bootcamps and online courses.
Traditional Degree Programs
A bachelor’s degree in fields like Statistics, Computer Science, or Data Science is often the first step. For more specialized roles, a master’s degree or even a Ph.D. is advisable.
Certifications and Bootcamps
Several organizations offer certifications in data analysis tools and techniques. Bootcamps are another fast-track option, focusing on practical skills over a short period.
Online Courses
Platforms like Coursera, Udemy, and edX offer a plethora of data analysis courses, allowing for flexible learning schedules and specialized skill acquisition.
Internships and Real-World Experience
Nothing replaces hands-on experience. Internships or entry-level jobs in data analysis can provide invaluable real-world exposure.
Continuous Learning and Specialization
The field of data analysis is continuously evolving, making it essential to stay updated through workshops, webinars, and further studies.
Soft Skills and Networking
Soft skills like communication and problem-solving are equally crucial in a data analysis career. Networking through industry events can also provide additional opportunities.
Becoming proficient in data analysis is a journey that involves academic preparation, hands-on experience, and continuous learning. From traditional degrees to modern bootcamps and online courses, multiple pathways can lead you to become an expert in the field. Alongside hard skills, honing your soft skills and building a strong professional network are integral parts of the career development process. So whether you’re just starting out or looking to advance your skills, the educational routes are diverse, accommodating various learning styles and career goals.
Future Outlook: Where Is Data Analysis Headed?
Now that we’ve covered the history, challenges, and educational pathways in data analysis, you may be curious about the future of this ever-evolving field. What are the emerging trends and technologies that are set to redefine data analysis?
The future of data analysis is promising and is likely to be shaped by trends such as artificial intelligence (AI), big data analytics, and real-time analysis. These advancements will allow for more accurate predictive models, automated decision-making, and quicker insights, transforming how we collect, analyze, and interpret data.
Curious about the next frontier in data analysis and how emerging technologies will change the game? Let’s unpack the trends that are set to revolutionize this field in the coming years.
Artificial Intelligence and Machine Learning
AI and machine learning algorithms will play a significant role in automating data collection and interpretation, making the process more efficient and accurate.
Big Data Analytics
The growth of big data will necessitate more advanced analytics tools, allowing professionals to sift through massive datasets to extract meaningful insights.
Real-Time Analysis
The demand for real-time analytics is growing. Whether it’s for monitoring health metrics or financial markets, instant data analysis will become increasingly critical.
Quantum Computing
Once it becomes more accessible, quantum computing has the potential to process complex calculations in fractions of the time currently required, reshaping data analysis methods.
Ethical Considerations
As data analysis grows more sophisticated, ethical concerns like data privacy and algorithmic bias will become increasingly important.
Industry-Specific Innovations
Each industry, from healthcare to marketing, will see specialized data analysis tools and methodologies emerge, tailored to their unique needs.
The future of data analysis is both exciting and complex, set to be influenced by technological advancements and emerging trends like AI, big data, and real-time analytics. These shifts promise to make data analysis more efficient, accurate, and timely, but they also introduce new challenges and ethical considerations. Keeping abreast of these changes will be crucial for anyone involved in data analysis, as the field continues to evolve at a rapid pace. Whether you’re a student, a professional, or simply someone interested in the world of data, there’s no doubt that data analysis will play an increasingly central role in our data-driven future.
Conclusion
From its historical roots to its current challenges and educational pathways, data analysis stands as a dynamic and crucial field. As we’ve explored, its future is teeming with possibilities and innovations, shaped by technologies like artificial intelligence, big data analytics, and real-time analysis. However, along with the advancements come challenges in data quality, security, and ethics that professionals and organizations must vigilantly address. The need for skilled analysts has never been greater, and the educational landscape offers multiple avenues for acquiring expertise.
If you’re interested in entering this field or are already a part of it, staying updated with the latest trends and challenges is not just beneficial—it’s essential. The world of data analysis is changing rapidly, and to remain relevant and effective, continuous learning and adaptability are key. Thank you for joining us on this comprehensive journey through the world of data analysis. We hope this blog post has provided you with valuable insights and a roadmap for what lies ahead in this fascinating field.
References
https://www.careerexplorer.com/careers/data-analyst/
https://en.wikipedia.org/wiki/Data_analysis
https://www.investopedia.com/terms/d/data-analytics.asp